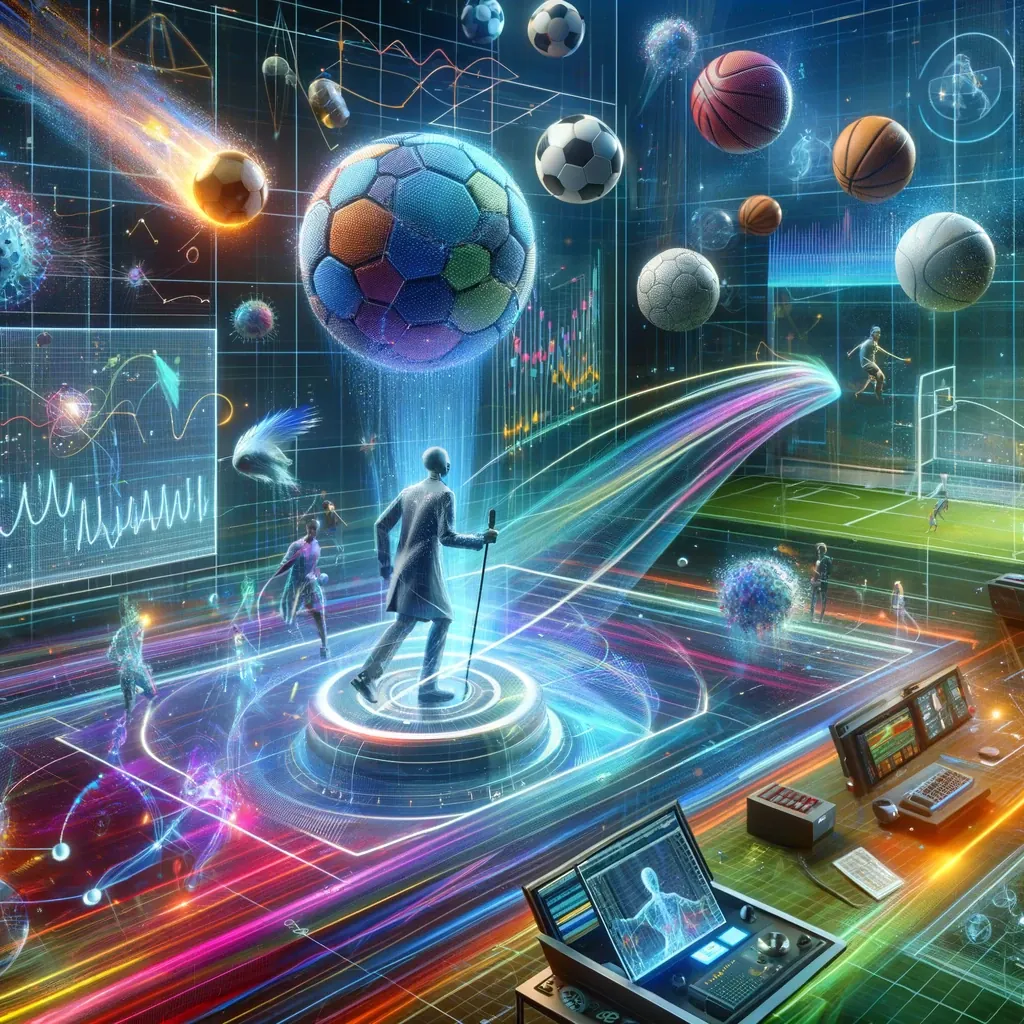
Challenges and Solutions in Spatial Dynamic Modeling
Explore the intricacies of spatial dynamic modeling with our comprehensive guide. Delve into the challenges of occlusions, varying lighting conditions, synthesized datasets, and background noise. Discover the innovative solutions developed by mlSpatial to revolutionize the analysis of object motion, velocity, and direction through cutting-edge machine learning models.
Spatial dynamic modeling is an intricate dance of mathematics, physics, and computer science, choreographed to understand and predict the motion and interaction of objects through space over time. This field’s applications are vast and varied, ranging from predicting weather patterns and understanding celestial dynamics to more terrestrial concerns like traffic flow management and the flight paths of drones. Yet, for all its utility, spatial dynamic modeling presents a slew of technical challenges, particularly when it comes to interpreting 2D images. In this article, we’ll explore these challenges, delve into the ingenious solutions developed by our team, and introduce how mlSpatial is revolutionizing this space.
Contents
- Deciphering Shadows: The Challenge of Occlusions
- Lighting the Way: Varying Lighting Conditions
- The Synthetic Conundrum: Synthesized Datasets
- A Symphony of Distractions: Background Noise
- mlSpatial: The Next Frontier
- The Road Ahead
- Further Reading
Deciphering Shadows: The Challenge of Occlusions
One of the first hurdles in spatial dynamic modeling from 2D images is occlusions. Objects can obscure each other, sometimes partially, sometimes entirely. Consider a soccer ball momentarily hidden under the shadow of a player; tracking its path becomes a puzzle of predicting its trajectory and speed without visual confirmation.
Innovative Solutions: Predictive Algorithms and Object Continuity
Our team approached this by developing predictive algorithms that estimate the object’s trajectory, speed, and even its interaction with other objects during occlusion. By analyzing the object’s motion before and after the occlusion, combined with the physics of its expected behavior, we can maintain a continuous track of the object’s movement, ensuring seamless spatial dynamic analysis.
Lighting the Way: Varying Lighting Conditions
Another significant challenge is the varying lighting conditions which can dramatically alter the appearance of objects, shadows, and the scene itself. From the glaring midday sun casting minimal shadows to the diffuse light of an overcast day, each condition requires a unique approach to accurately interpret images.
Adaptive Image Processing
To combat this, our team implemented adaptive image processing techniques that adjust parameters based on the lighting conditions detected in the image. This includes dynamic thresholding for object detection, contrast adjustments, and even the use of artificial intelligence to predict how lighting should affect the appearance of objects, improving the accuracy of our models under any lighting condition.
The Synthetic Conundrum: Synthesized Datasets
Creating realistic training datasets for machine learning models can be a formidable challenge. Real-world data is often messy, incomplete, or too variable, leading many researchers to use synthesized datasets. However, these datasets can sometimes stray too far from reality, leading models to learn patterns that don’t apply in real-world scenarios.
Bridging the Gap with Hybrid Approaches
Our solution was to create hybrid datasets that blend real-world data with synthesized elements. This approach allows our models to learn from the vast variability of real data while also benefiting from the controlled conditions of synthesized data. By carefully balancing these elements, we ensure our models are robust and versatile.
A Symphony of Distractions: Background Noise
Background noise, both literal and metaphorical, can significantly impair the accuracy of spatial dynamic models. From irrelevant objects moving in the background to fluctuations in environmental conditions, these distractions can lead models astray.
Focus Through Filtering
To address this, we’ve developed advanced filtering techniques that help isolate the signal from the noise. This involves a combination of spatial filters to remove unrelated background movements and temporal filters to smooth over erratic fluctuations in environmental conditions, ensuring our focus remains squarely on the object of interest.
mlSpatial: The Next Frontier
At mlSpatial, we specialize in ML models for analyzing spatial dynamics, leveraging photographic inputs to detect the spin, velocity, and direction of objects like balls. While we’ve made significant strides in sports analytics and beyond, our ambition doesn’t stop here. Our team is constantly exploring new frontiers, aiming to expand our technology’s applications to realms unimagined.
The Road Ahead
The journey of spatial dynamic modeling is filled with challenges, but each obstacle presents an opportunity for innovation. Through creativity, persistence, and a bit of technical wizardry, we’re not just overcoming these challenges—we’re setting new standards for what’s possible in the field. Whether it’s through refining our understanding of occlusions or pioneering new approaches to dealing with background noise, the future of spatial dynamics is bright, and at mlSpatial, we’re excited to be at the forefront of this exploration.
Further Reading
- Spatio-Temporal Data Mining: “A Survey of Problems and Methods,” covering applications in neuroscience, environmental science, and more ↗.
- Graph-based Deep Learning for Communication Networks: A comprehensive survey on solving networking-related problems with graph-based deep learning ↗.
- DG-STGCN: Dynamic Spatial-Temporal Modeling for Skeleton-based Action Recognition: Introduces a new framework for action recognition using dynamic graph convolutional networks ↗.